Brown CS Faculty Receive Brown’s OVPR Seed Award And Salomon Faculty Research Award Honors
- Posted by Jesse Polhemus
- on May 22, 2025
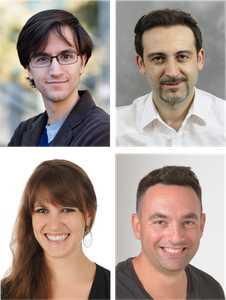
Brown CS faculty members Stephen Bach, Khosrowshahi University Professor of Computer Science Ugur Çetintemel, and Briger Family Distinguished Associate Professor of Computer Science Ellie Pavlick, along with their collaborators from other campus units, have just received Seed Awards from Brown University's Office of the Vice President for Research (OVPR) to help them compete more successfully for large-scale, interdisciplinary, multi-investigator grants. A new project by Brown CS faculty member Nikos Vasilakis has received Honorable Mention for Brown’s Richard B. Salomon Faculty Research Award, which supports excellence in scholarly work by providing funding for selected faculty research projects of exceptional merit, with preference given to junior faculty in the process of building their research portfolio.
Stephen Bach
Steve’s research (“AI-Enhanced Real-Time Selection Algorithms for Future Discoveries at the Large Hadron Collider and Beyond”) is led by Principal Investigator (PI) Loukas Gouskos, Assistant Professor of Physics. Steve is a Co-PI, along with Greg Landsberg, Thomas J. Watson, Sr. Professor of Physics.
“The Large Hadron Collider (LHC) generates an overwhelming volume of data,” the researchers explain, “far surpassing what tech giants like Amazon and Google manage, at a rate of around 40 million particle collision events every second, resulting in petabytes of raw data annually. To process this deluge of information, real-time decision-making algorithms must rapidly identify interesting events within microseconds. This SEED project aims to develop real-time selection algorithms using advanced Artificial Intelligence (AI) and Deep Learning (DL) methods to analyse this immense data load more efficiently than ever before. In a unique interdisciplinary collaboration between Brown University’s Physics and Computer Science departments, this project will develop AI models specifically optimized for the LHC’s Level-1 Trigger (L1T) system. The L1T operates under severe resource constraints, utilizing hardware such as field-programmable gate arrays (FPGAs) to make split-second decisions. These algorithms will significantly improve the detection of rare, beyond Standard Model (BSM) physics phenomena–potential discoveries that current methods might miss. The innovations developed here will not only push the boundaries of particle physics but also have transformative applications in fields such as astrophysics and medical diagnostics, where rapid, real-time data analysis is critical.”
Ugur Çetintemel
Ugur is Principal Investigator for his project (“An AI-Augmented Database System for Clinical Data Lakes”), with Zhicheng Jiao, Assistant Professor in Diagnostic Imaging, as Co-PI.
“Clinical data lakes,” the researchers explain, “integrate diverse data sources—such as electronic health records, medical imaging, lab results, and physician notes—offering immense potential for personalized medicine and evidence-based care. However, extracting value from these data lakes remains challenging due to their scale, heterogeneity, and the complexity of clinical information. Recent advances in AI, particularly large language models (LLMs), offer new possibilities for querying and interpreting such data, but their inherent unreliability and inefficiency limit adoption in critical healthcare settings.
This project aims to make AI-augmented processing of large clinical datasets both efficient and reliable, analogous to how traditional database systems revolutionized transactional workloads. We propose building a data processing engine that enables clinicians to query multi-modal clinical data lakes using natural language. By combining the flexibility of AI-driven interfaces with the robustness of database systems, this system will simplify and scale access to complex healthcare data. A central focus is the integration of reliability guardrails to manage LLM-related errors, hallucinations, and inconsistencies—ensuring safe deployment in sensitive clinical environments.”
Ellie Pavlick
Ellie is Principal Investigator for her project (“Comparing Logical Operators in Human and Artificial Intelligence”), with Roman Feiman, Thomas J. and Alice M. Tisch Assistant Professor of Cognitive and Psychological Sciences and Assistant Professor of Linguistics, as Co-PI.
“The goal of this interdisciplinary Seed proposal,” the researchers explain, “is to harness recent technical advances in AI, in particular, LLMs, in order to make theoretical contributions to understanding the nature of language and reasoning in humans. LLMs have received substantial attention and enthusiasm since the release of ChatGPT in 2022 due to their apparent ability to perform a wide range of tasks previously assumed to require human-level intelligence, such as reading advanced articles, producing cogent summaries, and writing computer code. Despite the impressiveness of LLMs as a technological and engineering feat, they have not yet contributed substantially to the study of language and cognition more broadly. This proposal considers the provocative hypothesis that, despite many obvious differences in their structure and development, LLMs and humans might invoke similar cognitive processes and adhere to similar principles and constraints, and thus, that understanding the mechanisms and representations that LLMs employ can provide new insights into the representations and mechanisms that underlie human thought.”
Ellie, Steve, and Ugur join numerous previous Brown CS recipients of OVPR Seed Awards, including (most recently) Nora Ayanian and Anna Lysyanskaya.
A full list of OVPR Seed Award recipients is available here.
Nikos Vasilakis
Nikos’ project (“Scaling Data Processing, for Everyone!”) responds to a worldwide need to accelerate data processing.
“Domain experts in most disciplines today rely on processing increasingly large and complex datasets,” he explains. “Accelerating such data processing is critical for getting insights on vaccine development, climate change, or ancient texts in minutes or hours—not weeks or years. Unfortunately, such acceleration today typically requires (1) expertise on scalable processing paradigms, the kind of expertise typically available only to PhDs in computer science; (2) burdensome effort to manually scale out each computation, even if the expertise is available; and (3) error-prone hand-scaling that might lead to incorrect results, even when such expert effort is expended.
This project aims at developing techniques, systems, and infrastructure for automatically and correctly scaling data processing that are field-agnostic, without the need for a developer. The proposed techniques combine automated pre-execution analysis of components to collect information about the computation, careful management of component effects during execution, and powerful just-in-time recoverability mechanisms rolling back optimizations that prove non-ideal—enabling novel synergistic techniques collectively termed opportunistic scaling. These techniques are anticipated to result in order-of-magnitude speedups on unmodified programs across environments while requiring no information from their users—experts and non-experts alike.”
A list of this year’s Richard B. Salomon Faculty Research Award recipients is available here.
For more information, please click the link that follows to contact Brown CS Communications Manager Jesse C. Polhemus.