A Physics-CS Collaboration: Detecting Satellite RFI
- Posted by Jesse Polhemus
- on Sept. 15, 2022
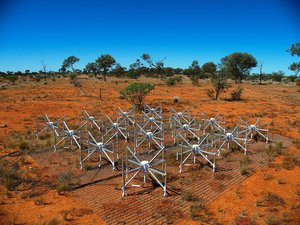
We're reposting this article with the kind permission of Brown University's Data Science Initiative.
Stephen Bach (Assistant Professor of Computer Science) and Jonathan Pober (Assistant Professor of Physics), along with colleagues at the University of Washington, have been awarded a Collaborative Research grant from the National Science Foundation, called RFI Detection Across Six Orders of Magnitude in Intensity: A Unifying Framework with Weakly Supervised Machine Learning.
Stephen Bach, a member and co-chair of DSI’s Campus Advisory Board, develops methods that drive down the labor costs of machine learning. Pober’s research focuses on observing neutral hydrogen in the cosmos--both improving observational techniques as well as the related physics of early universe galaxy formation. Their collaboration builds on work partly supported by a DSI seed grant awarded to Pober and his graduate student Joshua Kerrigan (Ph.D., ‘19).
Pober’s research depends on data collected by the Murchison Widefield Array (right), a low-frequency radio telescope in Australia. Brown has recently taken over as the lead organization for US-based researchers working on the MWA. Pober’s collaboration with Bach addresses a growing problem for ground-based astronomy caused by the Starlink satellites being launched by SpaceX (along with those planned by other companies). These cause problems for optical astronomy, due to their brightness and numbers, but are also a concern for radio astronomy, as radio frequency interference (RFI) from human-made satellites complicates detection of cosmological signals, especially faint ones. (For more information, see here and here.)
The goal of the current project is to develop machine learning techniques to remove human-generated radio transmissions from MWA data. Pober and Bach are seeking to build on existing techniques for detecting RFI in order to develop a single technique that can identify very weak as well as stronger RFI (and everything in between, which can vary over six orders of magnitude of signal strength). An example of the very weak RFI that needs to be identified and distinguished from cosmological signals would be a digital television station in Indonesia bouncing off an orbiting satellite and reflecting into the array in Western Australia. This sort of weak signal will become more prevalent as more satellites are launched.
Pober says, “I really do think this is a great example of the kind of research that a Data Science Institute [at Brown] could catalyze. The NSF program is called Spectrum and Wireless Innovation enabled by Future Technologies (SWIFT), and it seemed like the perfect program for our particular skills: solving problems related to the use of the electromagnetic spectrum with explicitly interdisciplinary teams developing ‘future technologies’ (which specifically included machine learning). It's absolutely not a program any one of us could have competed for on our own, but thanks to the connections we had already established, we were able to put together what was obviously a competitive proposal!” DSI is excited to have been able to support a small part of the work that led to this collaboration, since fostering this type of data-focused research collaboration is an important part of our mission on campus.
Photo credit: Natasha Hurley-Walker, CC BY-SA 3.0 <https://creativecommons.org/licenses/by-sa/3.0>, via Wikimedia Commons
For more information, click the link that follows to contact Brown CS Communications Manager Jesse C. Polhemus.