Brown CS Receives Two Awards At SIGMOD 2019
- Posted by Jesse Polhemus
- on Aug. 23, 2019
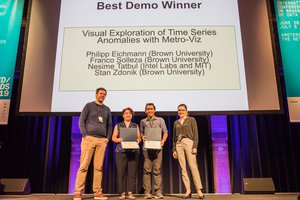
Click the links that follow for more news about Nathaniel Weir, Stan Zdonik, and other recent accomplishments by our faculty, students, and alums.
This year's ACM SIGMOD (Association for Computing Machinery's Special Interest Group on Management of Data) conference was held in Amsterdam, and members of the Brown CS community returned from the event with two notable awards. PhD candidates Philipp Eichmann, Franco Solleza, and Junjay Tan; alum Nesime Tatbul; and Professor Stan Zdonik received the Best Demonstration Award and alums Nathaniel Weir and P. Ajie Utama earned Second Place in the Undergraduate category of the Student Research Competition.
Philipp, Franco, Junjay, Nesime, and Stan's research ("Visual Exploration of Time Series Anomalies with Metro-Viz") presents a novel data visualization solution for exploring the results of time series anomaly detection systems. "When anomalies are reported," they explain, "there's a need to reason about the results, and so we introduce Metro-Viz, a visual tool to assist data scientists in performing this analysis." Metro-Viz offers a rich set of interaction features, including comparative analysis and what-if testing, backed by data management strategies specifically tailored to the workload. In their demo, they showed the tool in action via multiple time series datasets and anomaly detectors.
The project's web site is here, and the researchers are recognized in the first three minutes of the award session video here.
Nathaniel and P. Ajie's research ("Bootstrapping an End-to-End, Cross-Domain NLI for Databases") offers an end-to-end Natural Language Interfaces for Databases (NLIDB) framework in which a neural translation model is trained for any new database schema with minimal manual overhead. "The ability to extract insights from data," they write, "is critical for decision making. Intuitive natural language interfaces to databases provide non-technical users with an effective way to formulate complex questions and information needs efficiently and effectively." In addition to being the first off-the-shelf, neural machine translation based system of its kind, their project's other contribution is its use of a synthetic training set generation pipeline used to bootstrap a translation model without requiring manually curated data. In experiments, they show that their system can achieve competitive performance on the recently released benchmarks for nl-to-sql translation.
For more information, click the links that follow to contact Brown CS Communication Outreach Specialist Jesse C. Polhemus.