Brown CS PhD Student Victor Ojewale And Collaborators Receive An IEEE SATML Distinguished Paper Award
- Posted by Jesse Polhemus
- on May 17, 2024
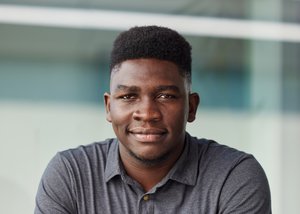
Held in Toronto, Canada, last month, the IEEE Conference on Secure and Trustworthy Machine Learning (IEEE SATML) focuses on expanding on the theoretical and practical understandings of vulnerabilities inherent to ML systems, exploring the robustness of ML algorithms and systems, and aiding in developing a unified, coherent scientific community which aims to build trustworthy ML systems. The event’s organizers recognized only two papers with their Distinguished Paper Award, and new research by Brown CS PhD student Victor Ojewale was one of them.
Victor is advised by faculty member Suresh Venkatasubramanian of Brown CS, Brown University’s Data Science Institute, and Brown’s Center for Technological Responsibility, Reimagination, and Redesign. His work (“AI auditing: The Broken Bus on the Road to AI Accountability”) is a collaboration with Abeba Birhane (Mozilla Foundation and Trinity College Dublin), Ryan Steed (Carnegie Mellon University), Briana Vecchione (Data & Society), and Inioluwa Deborah Raji (Mozilla Foundation and University of California, Berkeley).
“One of the most concrete measures to take towards meaningful AI accountability,” the authors explain, “is to consequentially assess and report the systems’ performance and impact. However, the practical nature of the ‘AI audit’ ecosystem is muddled and imprecise, making it difficult to work through various concepts and map out the stakeholders involved in the practice.”
In their paper, the researchers first taxonomize current AI audit practices as completed by regulators, law firms, civil society, journalism, academia, and consulting agencies. Next, they assess the impact of audits done by stakeholders within each domain. Spurred by their finding that only a subset of AI audit studies translate to desired accountability outcome, they assess and isolate practices necessary for effective AI audit results, articulating the observed connections between AI audit design, methodology, and institutional context on its effectiveness as a meaningful mechanism for accountability.
“We wrote our paper,” Victor tells us, “because audits are critical to figuring out whether AI systems work and how they can be improved. We found that audits by journalists such as those in ProPublica and The Markup are particularly good, largely because they have a comprehensive methodology for performing audits. These practices go far beyond more ‘academic’ audits, and have helped push AI systems to be better.”
"As more organizations – private, and public – begin to deploy automated decision systems,” says Suresh, “it becomes really important that we build effective systems of accountability for them. Victor and his colleagues' work provides crucial insights for all of us seeking to ensure that AI provides benefits for – and does not harm – all of us.”
For more information, click the link that follows to contact Brown CS Communications Manager Jesse C. Polhemus.