DIMACS Workshop Bridges LLMs And Game Theory
- Posted by Jesse Polhemus
- on Nov. 14, 2023
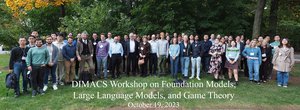
by Deni Goktas, Brown CS PhD Candidate
The DIMACS Workshop on Foundation Models, Large Language Models (LLMs), and Game Theory, held at Rutgers University on October 19 and 20, 2023, marked the first of many foreseeable steps towards advancing a research initiative at the intersection of these topics. With a notable shift towards generative AI—models trained on extensive data to generate content adaptable to a myriad of downstream tasks—the workshop aimed to delve into the role that game theory, a mathematical subfield of economics, could play in contributing to these developments and pondered the reverse impact that generative AI can have on game theory.
Organized by Professor Amy Greenwald and PhD student Deni Goktas, together with researchers from Rutgers University and IBM, the workshop featured a series of research talks by academics and industry professionals. It also included rump and poster sessions showcasing preliminary directions, many presented by students. The workshop concluded with a panel and breakout sessions to outline future directions based on the research questions prompted by the workshop.
Game theory models the strategic interaction of agents, historically people or groups of people, with incentives. The multi-agent systems community has long taken the view that game theory is likewise suitable to model interactions among AI agents. To this end, the first day commenced with an engaging keynote by Constantinos Daskalakis of MIT, focusing on the prerequisites for training strategic foundation models, which raises an interesting question of how to endow foundation models with appropriate incentives, a problem closely related to value alignment. This keynote initiated a session on how the tools of game theory might be applied to enhance foundation models.
The afternoon keynote talk, which focused on the possibilities and limitations of large language models, was given by Kathy McKeown of Columbia University. Her talk was followed by a session on how foundation models can potentially be used to solve game theory problems. For example, Ph.D. student Athul Paul Jacob discussed his work on incorporating LLM technology into strategic AI agents capable of playing the board game Diplomacy, a game of strategy that requires negotiation in natural language. These talks improved our understanding of how foundation models can be employed to enhance game solvers, which at present seem to require separate modules for language and reasoning. (There was extensive discussion at the end of the workshop as to whether this separation would remain necessary.)
Subsequent to these two sessions, researchers participated in rump and poster sessions presenting burgeoning findings at the crossroads of foundation models, LLMs, and game theory. Among other results, the posters presented novel neural architectures for building foundation models that solve games and novel LLM-based multiagent systems for tackling critical tasks such as causal reasoning. The day concluded with a dinner, where guest of honor Michael Littman—Brown CS faculty member and Division Director at the National Science Foundation—delivered a talk on the limits and possibilities of AI in education.
The momentum from the first day carried into the next, with a keynote by John Horton of MIT, who explored the role of LLMs in behavioral economics, by asking whether LLMs might be capable of serving the role of human subjects in some capacity, enabling pilot studies, full transparency, easy replication, and fast iteration. The day continued to uncover the vast potential at the intersection of game theory and foundation models, covering topics ranging from generating reward feedback using foundation models, thus scaling human feedback; to automated test case generation and self-improving code generation; to novel economic models for pricing foundation models. The workshop concluded with a panel discussion on the impact of foundation models and LLMs on society, featuring experts from Carnegie Mellon, MIT, University of British Columbia, and Rutgers.
The DIMACS Workshop on Foundation Models, Large Language Models, and Game Theory was more than just an academic exchange; it acted as a foundation for future collaborations and a catalyst for innovation at the junction of generative AI and game theory. The discussions, presentations, and interactions among the attendees not only highlighted the current state of the art, but also mapped out a trajectory for future explorations. The application of game-theoretic models and tools in the realm of foundation models is set to unveil new dimensions in AI, pushing the field towards unexplored territories.
If you missed the workshop, videos of the presentations are available on the workshop website.