Ellie Pavlick Joins Brown CS As Assistant Professor
- Posted by Jesse Polhemus
- on June 20, 2017
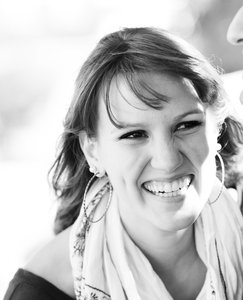
Brown University's Department of Computer Science (Brown CS) has just added another new faculty member: Ellie Pavlick is joining us as Assistant Professor in the summer of 2018. “I’m still interested in economics,” she says (Ellie has a BA in that field and a BM in saxophone performance), “but I felt like it was much messier.” Maybe so, but after sitting down to talk for an hour, it’s clear that Pavlick in no way finds messiness to be a source of discomfort. An expert in crowdsourcing and paraphrasing, studying language in all its complexity has allowed her to use what she calls a problem-driven approach, combining sprawling interests in a world where no two words mean the same thing.
If you think that description sounds like a good fit for Brown University, you’re not alone. “I love the broad interests at Brown,” says Ellie. “Understanding language is a deep, difficult interdisciplinary problem. Academia lets you play that long game. And getting to work with students means less uniformity. Each student has their own interests, so they help you get involved with a lot of different things.”
Pavlick’s own involvement with computer science came surprisingly late. “I’ve always been interested in multiple things,” she says. “I find it hard to feel fulfilled with just one area. I think if I’d really had a chance to explore CS in high school, I would’ve gone into it sooner because it provides this common skill set that can be applied to so many areas.” During undergraduate studies at Johns Hopkins, using LaTeX and MATLAB sparked Ellie’s interest in the field. Soon after, participation in Women in CS events helped her find an advisor and start new research projects. After graduation, when one of her professors transferred to University of Pennsylvania, she followed, giving up plans for a Master’s in favor of a PhD.
Natural language processing and crowdsourcing appealed to Pavlick from the beginning: “People speak and understand human language, not programming languages, which is what computers quote-unquote ‘speak’. I find it really exciting: our language is very complex, and it’s not designed for talking to computers. It’s full of not just commands but implications and feelings. Can we get computers to unpack all of that implicit meaning?”
Driven by my own interests, I pull Ellie into a side discussion of writerly craft and whether any two words can mean exactly the same thing. Does she see herself as a writer? “Definitely. I like working with language because it’s an incredible mix of structure and freedom. I used to think I’d end up as more of an artsy person...even when I write up research, which I guess is technical writing, I really enjoy it and probably overthink it. People can be very imprecise with language, but we can also be very particular about how we say what we say. That has gotten less attention from an AI perspective. People write and speak with the expectation that the reader or listener is a human, so we use a lot of hints and cues to indicate our opinions and impressions and these are completely lost on computers.”
And that person-to-computer communication, ultimately at a seamless level, is coming, Ellie thinks, but the timeframe is tough to estimate. “We’ve made incredible progress in AI on things that look like language understanding,” she says, “but the models are shallow. As a field, we’ve just started a conversation about modeling intents and goals, but computers haven’t even begun to understand how human language expresses differing personalities and similar but distinct goals.”
The way to get there, Pavlick says, is to make computer science even more interdisciplinary than it already is: “We can’t just keep cranking away at what we’re doing. Natural language processing is a young field, and it’s hard to predict what kind of knowledge is needed next. People coming into CS with different interests and perspectives provides the raw ingredients we need for progress, for the next advance.”
And having a different methodology is equally important. “One question I get a lot,” Ellie says, “is if deep learning will solve all of our problems. ‘Isn’t language solved?’ As computer scientists, we sometimes fall into this love affair with a certain method. We find an exciting model and start looking for things to apply it to, and then want to report on what’s going well. What I like about Brown is the problem-driven approach, which drives you to collaborate and forces you to question and to choose the best methods from a large, interdisciplinary set of possible methods. Research should be based around the things we don’t know how to do.”
For more information, click the link that follows to contact Brown CS Communication Outreach Specialist Jesse C. Polhemus.