Erik Sudderth And Collaborators Advance Seismic Monitoring And Nuclear Non-Proliferation, Earning A Top Prize In Bayesian Analysis
- Posted by Jesse Polhemus
- on Oct. 13, 2015
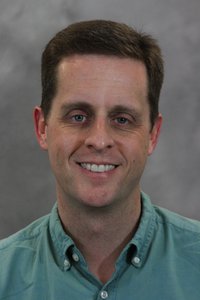
Few things are less abstract than an earthquake or more important than nuclear non-proliferation, and one of the top awards in Bayesian analysis highlights how complex statistical models can be used to solve problems that go far beyond theory. The International Society for Bayesian Analysis (ISBA, which sponsors leading journals and conferences) presents the Mitchell Prize in annual recognition of an outstanding paper that uses Bayesian analysis to solve an important applied problem.
This year, Professor Erik Sudderth of Brown University’s Computer Science Department and his collaborators (Professor Stuart Russell of University of California, Berkeley and Nimar S. Arora of Bayesian Logic, Inc. and BetaZi) have received the award for their research in seismic monitoring. Their work (“NET-VISA: Network Processing Vertically Integrated Seismic Analysis”), which was also highlighted (“Global Seismic Monitoring: A Bayesian Approach”) at the Twenty-Fifth Conference on Artificial Intelligence (AAAI-11), involves automated processing of multiple seismic signals, a central problem in both geophysics and nuclear treaty verification.
“Around the globe,” Erik explains, “one of the most important mechanisms for nuclear non-proliferation is the global sensor network that identifies seismic events and their locations. As part of the Comprehensive Nuclear Test Ban Treaty, it’s a key tool to ensure that nuclear weapons aren’t being tested. Unfortunately, identifying true seismic events at a global scale is a complex task. Our Bayesian seismic monitoring system rigorously models geophysical uncertainty to avoid missed events. In the end, we reduce them by 60% compared to a highly advanced baseline system, and we even find events missed by expert human analysts. As worldwide interest in mitigating the risks of nuclear proliferation grows, improving the accuracy of seismic signal processing becomes more urgent every day. We’re tremendously proud to use statistical machine learning to contribute to an area of global importance.”