Language Representation and Inference
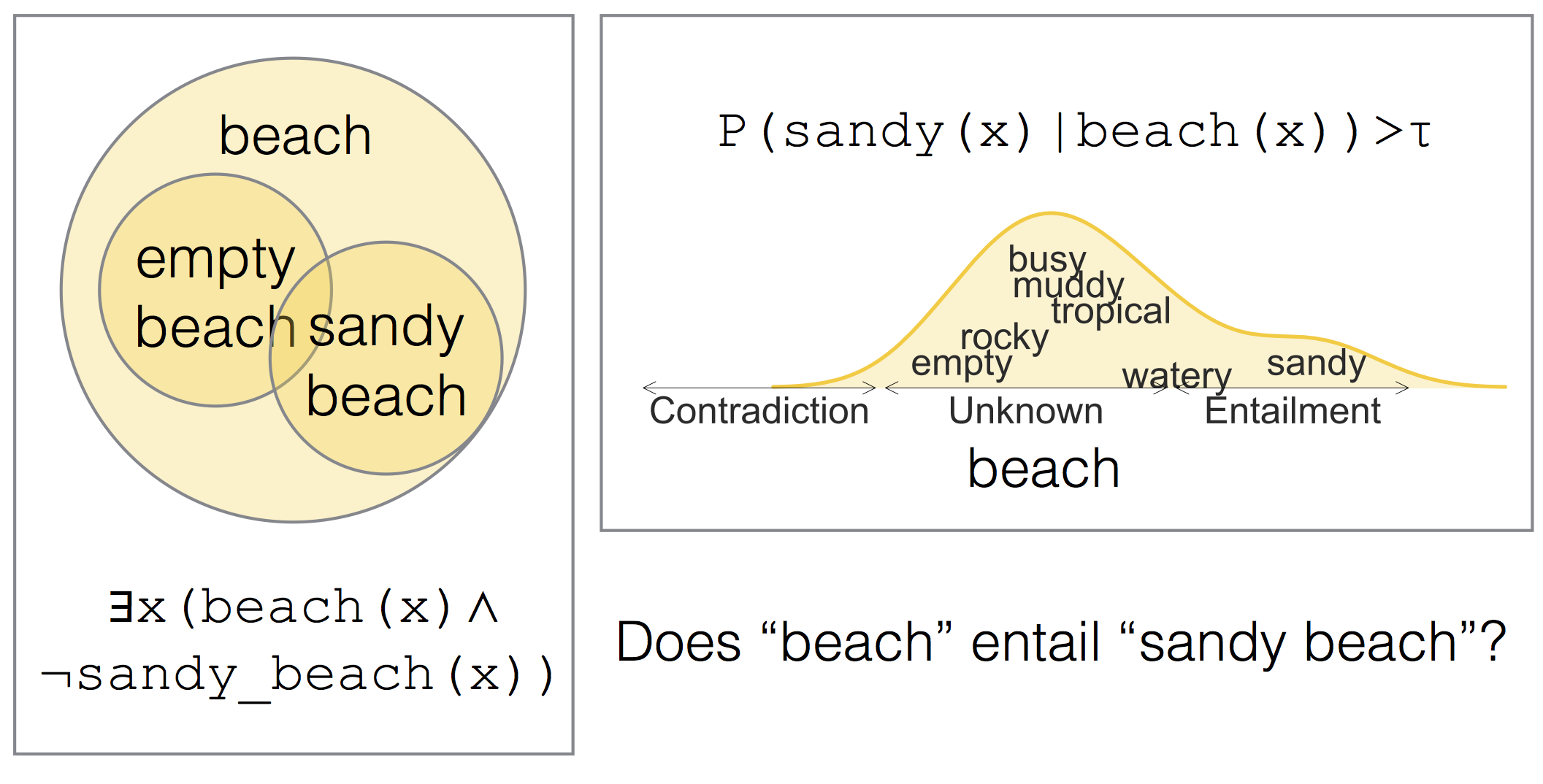
Natural language is an incredibly efficient way of representing information. Humans can express seemingly arbitrarily precise facts about the world: "The Bloomberg dollar index reversed earlier gains to trade 0.6 percent lower at 13:35 p.m. in New York and continued to tread water even as the 10-year Treasury yield climbed to 2.90 percent, a fresh four-year high."* We can also express highly complex scenarios using the vaguest of language: "That's just Alice being Alice."
There seems to be some kind of magic that happens in humans' heads that allows us to conjure up a staggering amount of information in order to make inferences: not just information about language and grammar, but about the world and the experience of living in it, and about the speakers and listeners, their assumptions, and their intents. What, exactly, is happening? Can it be codified in a way that computers can understand?
The main questions I am interested in in this area are questions about representation and learning. What is "common sense" and what does it "look like"? How do humans represent all of our knowledge about the world within our fixed-sized brains, and how do we access it in order to make inferences seemingly instantaneously? How to we build these representations through the experience of living rather than through supervised training? And of course, how can we make the answers to these questions play well within a computational system?
Here are some of my publications related to the topic. This will be growing soon with the help of my amazing to-be students!
- Compositional Lexical Entailment for Natural Language Inference. PDF Slides
- So-Called Non-Subsective Adjectives. PDF
- Most babies are little and most problems are huge: Compositional Entailment in Adjective Nouns. PDF Data
- Identifying 1950s American Jazz Musicians: Fine-Grained Isa Extraction via Modifier Composition. PDF
- Tense Manages to Predict Implicative Behavior in Verbs PDF