 |
 |
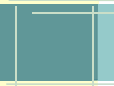 |
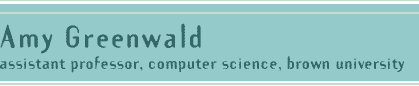 |
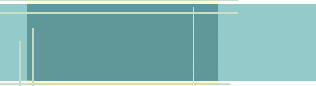 |
|
-
In this talk, we define a general class of no-regret learning
algorithms, called no-Φ-regret learning algorithms, and a a class
of game-theoretic equilibria, called Φ-equilibria, we show that
for all φ ε Φ, the empirical distribution of play of
no-φ-regret algorithms converges to the set of Φ-equilibria.
-
[ps]
[pdf]
- Presented at
Caltech, October 2003.
[abstract]
- Presented at
COLT, August 2003.
[abstract]
- Joint work with
Amir Jafari.
- This work identifies properties of learning algorithms
which give rise to equilibrium outcomes in network games.
- New York University
Dissertation Defense & Job Talk, May 1999.
- [ps]
[pdf]
[abstract]
- This research includes both negative and positive results on
learning in the Santa Fe Bar Problem.
- Presented at the The Stony Brook Game Theory Festival: Workshop on Interactive Dynamics and Learning,
SUNY Stony Brook, July 1998.
- Joint work with Bud Mishra and Rohit Parikh.
- [ps]
[pdf]
[abstract]
- This presentation provides an introduction to the connection
between learning, game theory, and networks.
- DARPA
Graduate Student Workshop, Washington, DC, July 1998.
- [ps]
[pdf]
[abstract]
|
|
|
|
|