CSCI2951-B Data-Driven Vision and Graphics
Spring 2013, MWF 11:00 to 11:50, CIT 506.
Instructor: James Hays
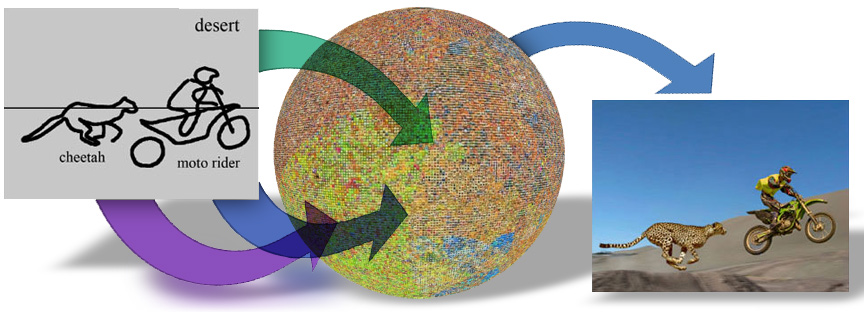
Course Description
Course Catalog EntryThis graduate seminar course investigates current research topics in image-based, data-driven graphics and vision. We will examine data sources, features, and algorithms useful for understanding and manipulating visual data. We will pay special attention to methods that harness large-scale or Internet-derived data. Vision topics such as scene understanding and object detection will be linked to graphics applications such as photo editing and image-based rendering. These topics will be pursued through independent reading, class discussion and presentations, and state-of-the-art projects.
The goal of this course is to give students the background and skills necessary to perform research in image-based graphics and vision. Students should understand the strengths and weaknesses of current approaches to research problems and identify interesting open questions and future research directions. Students will hopefully improve their critical reading and communication skills, as well.
Course Requirements
Reading and Summaries
Students will be expected to read one or two papers for each class. For every assigned paper, students must write a two or three sentence summary and identify at least one question or topic of interest for class discussion. Interesting topics for discussion could relate to strengths and weaknesses of the paper, possible future directions, connections to other research, uncertainty about the conclusions of the experiments, etc. Reading summaries must be posted to the class blog by 11:59pm the day before each class. Feel free to reply to other comments on the blog and help each other understanding confusing aspects of the papers. The blog discussion will be the starting point for the class discussion. If you are presenting you don't need to post a summary to the blog.Class participation
All students are expected to take part in class discussions. If you do not fully understand a paper that is OK. We can work through the unclear aspects of a paper together in class. If you are unable to attend a specific class please let me know ahead of time (and have a good excuse!).Presentation(s)
Depending on enrollment, students will lead the discussion of one or two papers during the semester. Ideally, students would implement some aspect of the presented material and perform experiments that help understand the algorithms. Presentations and all supplemental material should be ready one week before the presentation date so that students can meet with the instructor, go over the presentation, and possibly iterate before the in-class discussion. For the presentations it is fine to use slides and code from outside sources (for example, the paper authors) but be sure to give credit.Semester projects
Students are expected to complete a state-of-the-art research project on topics relevant to the course. Students will propose a research topic part way through the semester. After a project topic is finalized, students will meet occasionally with the instructor to discuss progress. Students will present their progress on their semester project twice during the course and the course will end with final project presentations. Students will also produce a conference-formatted write-up of their project. Projects will be published on the this web page. The ideal project is something with a clear enough direction to be completed in a couple of months, and enough novelty such that it could be published in a peer-reviewed venue with some refinement and extension.Prerequisites
Strong mathematical skills (linear algebra, calculus, probability and statistics) and previous imaging (graphics, vision, or computational photography) courses are needed. It is strongly recommended that students have taken one of the following courses (or equivalent courses at other institutions):- CSCI 1230, Introduction to Computer Graphics
- CSCI 1290, Computational Photography
- CSCI 1430, Introduction to Computer Vision
- CSCI 2240, Interactive Computer Graphics
- ENGN 1610, Image Understanding
Textbook
We will not rely on a textbook, although the free, online textbook "Computer Vision: Algorithms and Applications" by Richard Szeliski is a helpful resource.Grading
Your final grade will be made up from- 20% Reading summaries posted to class blog
- 20% Classroom participation and attendance
- 20% Research presentation(s)
- 40% Semester project
Office Hours:
James Hays, Monday and Wednesday 2:00-3:00pm, CIT 375Schedule
Date | Paper | Paper, Project page, and Material | Presenter |
W, Jan 23 | Introduction | James | |
F, Jan 25 | The state of vision and graphics | James | |
M, Jan 28 | 80 million tiny images: a large dataset for non-parametric object and scene recognition. A. Torralba, R. Fergus, W. T. Freeman. IEEE Transactions on Pattern Analysis and Machine Intelligence, vol.30(11), 2008. | pdf, project page | James |
W, Jan 30 | Object recognition from local scale-invariant features, David Lowe, ICCV 1999. | pdf, project page | James |
F, Feb 1 | Video Google: A Text Retrieval Approach to Object Matching in Videos. Sivic, J. and Zisserman, A. Proceedings of the International Conference on Computer Vision (2003) | pdf, project page | James |
optional reading | Robust wide baseline stereo from maximally stable extremal regions. J. Matas, O. Chum, U. Martin, and T Pajdla. Proceedings of the British Machine Vision Conference, 2002. | ||
M, Feb 4 | Histograms of Oriented Gradients for Human Detection. Navneet Dalal and Bill Triggs. In Proceedings of IEEE Conference Computer Vision and Pattern Recognition, 2005. | James | |
W, Feb 6 | Beyond Bags of Features: Spatial Pyramid Matching for Recognizing Natural Scene Categories. S. Lazebnik, C. Schmid, and J. Ponce, CVPR 2006. | pdf, project page | James |
F, Feb 8 | No Classes - Brown closed due to blizzard | ||
M, Feb 11 | Scene Completion Using Millions of Photographs. James Hays, Alexei A. Efros. ACM Transactions on Graphics (SIGGRAPH 2007). August 2007, vol. 26, No. 3. | project page | James |
W, Feb 13 | Matching Local Self-Similarities across Images and Videos. Eli Shechtman and Michal Irani. IEEE Conference on Computer Vision and Pattern Recognition 2007 (CVPR'07) | project page | James |
F, Feb 15 | SUN Database: Large-scale Scene Recognition from Abbey to Zoo. J. Xiao, J. Hays, K. Ehinger, A. Oliva, and A. Torralba. IEEE Conference on Computer Vision and Pattern Recognition (CVPR2010). | project page | James |
M, Feb 18 | No Classes | ||
W, Feb 20 | What makes Paris look like Paris? Carl Doersch, Saurabh Singh, Abhinav Gupta, Josef Sivic, and Alexei A. Efros. Siggraph 2012. | project page | Hari |
F, Feb 22 | Learning to predict where humans look. T. Judd, K. Ehinger, F. Durand, and A. Torralba. IEEE International Conference on Computer Vision (ICCV), 2009. | project page | Hua |
M, Feb 25 | ImageNet: A Large-Scale Hierarchical Image Database. J. Deng, W. Dong, R. Socher, L.-J. Li, K. Li and L. Fei-Fei. IEEE Computer Vision and Pattern Recognition (CVPR), 2009 | pdf,project page | Zach |
W, Feb 27 | How do humans sketch objects? Mathias Eitz, James Hays, and Marc Alexa. Siggraph 2012. | project page | Jeroen |
F, Mar 1 | Recognizing Jumbled Images: The Role of Local and Global Information in Image Classification. Devi Parikh. ICCV 2011 | Ryan | |
M, Mar 4 | Micro Perceptual Human Computation for Visual Tasks. Yotam Gingold, Ariel Shamir, Daniel Cohen-Or. ACM Transactions on Graphics (ToG) 2012 | project page | Xiaofeng |
W, Mar 6 | Project Status Updates. | Everyone | |
F, Mar 8 | Project Status Updates. | Everyone | |
M, Mar 11 | Texture Optimization for Example-based Synthesis. Vivek Kwatra, Irfan Essa, Aaron Bobick and Nipun Kwatra. Siggraph 2005 | project page | Vibhu |
W, Mar 13 | PatchMatch: A Randomized Correspondence Algorithm for Structural Image Editing. Connelly Barnes, Eli Shechtman, Adam Finkelstein, and Dan B Goldman. Siggraph 2009. | project page | Greg |
F, Mar 15 | Image Melding: combining inconsistent images using patch-based synthesis. Soheil Darabi, Eli Shechtman, Connelly Barnes, Dan B Goldman, Pradeep Sen. Siggraph 2012. | project page | Jeroen |
M, Mar 18 | Internal Statistics of a Single Natural Image. Maria Zontak and Michal Irani. CVPR 2011. | pdf, project page | Hua |
W, Mar 20 | Super-resolution from Internet-scale Scene Matching. Libin "Geoffrey" Sun and James Hays. IEEE International Conference on Computational Photography (ICCP 2012). | project page | Krishna |
F, Mar 22 | Video Deblurring of Hand-held Cameras using Patch-based Synthesis. Sunghyun Cho, Jue Wang, and Seungyong Lee. Siggraph 2012. | project page | Hobart |
M, Mar 25 | No Classes | ||
W, Mar 27 | No Classes | ||
F, Mar 29 | No Classes | ||
M, Apr 1 | Eulerian Video Magnification for Revealing Subtle Changes in the World. Hao-Yu Wu, Michael Rubinstein, Eugene Shih, John Guttag, Fredo Durand, William T. Freeman. Siggraph 2012. | project page | Vibhu |
W, Apr 3 | CG2REAL. M.K. Johnson, K. Dale, S. Avidan, H. Pfister, W.T. Freeman and W. Matusik. IEEE Trans. on Visualization and Computer Graphics 2010. | project page | Chao |
F, Apr 5 | LabelMe: a Database and Web-based Tool for Image Annotation. B. C. Russell, A. Torralba, K. P. Murphy, W. T. Freeman. International Journal of Computer Vision, 2008. | pdf, project page | Xiaofeng |
M, Apr 8 | Photo Clip Art. Jean-François Lalonde, Derek Hoeim, Alexei A. Efros, Carsten Rother, John Winn and Antonio Criminisi. ACM Transactions on Graphics (SIGGRAPH 2007). | project page | Valay |
W, Apr 10 | Project Status Updates. | Everyone | |
F, Apr 12 | Project Status Updates. | Everyone | |
M, Apr 15 | Sketch2Photo: Internet Image Montage. ACM SIGGRAPH ASIA 2009, ACM Transactions on Graphics. Tao Chen, Ming-Ming Cheng, Ping Tan, Ariel Shamir, Shi-Min Hu. | project page | Yipin |
W, Apr 17 | Transfusive Image Manipulation. Kaan Yücer, Alec Jacobson, Alexander Hornung, Olga Sorkine. Siggraph Asia 2012 | project page | Hobart |
F, Apr 19 | Describing Objects by Their Attributes. A. Farhadi, I. Endres, D. Hoiem, and D.A. Forsyth. CVPR 2009 | project page | Zach |
M, Apr 22 | SUN Attribute Database: Discovering, Annotating, and Recognizing Scene Attributes. Genevieve Patterson and James Hays. CVPR 2012 | project page | Hari |
W, apr 24 | Relative Attributes. Devi Parikh and Kristen Grauman. ICCV 2011. | project page | Krishna |
F, Apr 26 | Ground-truth dataset and baseline evaluations for intrinsic image algorithms. R. Grosse, M.K. Johnson, E.H. Adelson and W.T. Freeman. ICCV 2009 | project page | Valay |
M, Apr 29 | User Assisted Intrinsic Images. Adrien Bousseau, Sylvain Paris, Fredo Durand. Siggraph Asia 2009 | project page | Ryan |
W, May 1 | Estimating Intrinsic Images from Image Sequences with Biased Illumination. Yasuyuki Matsushita, Stephen Lin, Sing Bing Kang, and Heung-Yeung Shum. 2004 | project page | Greg |
F, May 3 | Coherent Intrinsic Images from Photo Collections. Pierre-Yves Laffont, Adrien Bousseau, Sylvain Paris, Fredo Durand, and George Drettakis. Siggraph Asia 2012. | project page | Yipin |
M, May 6 | Rich Intrinsic Image Decomposition of Outdoor Scenes from Multiple Views. Pierre-Yves Laffont, Adrien Bousseau, George Drettakis. TVCG 2013. | project page | Chao |
Thursday, May 16 2pm (final exam slot) | Final Project Presentations | Everyone |